This article is a guest post written by Adii Pienaar, Co-Founder and CEO at Cogsy, an inventory management software platform.
During the pandemic, ecommerce experienced 10 years of growth in roughly three months. For a moment (the one where seemingly everyone was out of stock), this trend felt like it would last forever.
Before the pandemic, stockouts cost brands ~$1 trillion annually. But during the 2020 holiday season, stockouts were up 360% from January 2019, costing brands even more.
Many retailers responded by getting more aggressive with their inventory management and inventory forecasting. The goal was to have enough stock to fulfill this unprecedented demand.
This, of course, backfired.
Seemingly overnight, the world returned to a resemblance of normal (whatever that means). And coupled with rising inflation, demand for consumer goods dropped. (Ecommerce has since returned to its pre-pandemic growth trajectory.)
This has left retailers – big names like Nike, Target, and Allbirds included – scrambling to offload excess inventory and missing Wall Street projections as a result.
But is this overstock simply consequences of bad inventory bets? Not exactly. A few false forecasting assumptions are largely to blame.
5 common inventory forecasting misconceptions
Generally speaking, five false forecasting assumptions lead to really expensive, totally preventable inventory mistakes (like stockouts and excess inventory). And they’re only exacerbated when things outside the retailer’s control (like the supply chain) go wrong.
Misconception 1: Good data inherently means good forecasts
"A [forecasting] system is only as good as the data that's being put in," Archie Durfee, Associate Director of Supply Chain at Ro, recently said on The Checkout podcast. Most retailers operate under a similar belief.
But while we can probably all agree that not all data is created equal, having a few good data points doesn't inherently mean your forecasts will be reliable.
That's because the problem isn't about data being "good" or "bad." It's about retail brands not having all their data in one place.
For instance, you might manage your cash flow in a tool like Settle, production in Anvyl, fulfillment in ShipBob, and returns in Loop. And your ERP might act as a sort of middleware, connecting most of those data sources.
But no ERP is tech stack androgynous. Meaning, with the proliferation of sales channels and new operational tools, brands will need to invest in developing custom integrations to get the rest of their operational data into their ERP. This, of course, is not a viable pursuit for most brands.
As a result, reliable data remains hard to come by.
When you can't programmatically pull data from a single source of truth, you're left with data gaps. At that point, it doesn't matter if you have a few really, really good data points.
Sure, with spreadsheets, you can finagle whatever data points you find to fill these gaps. But similar to shoving a round peg in a square hole, the final forecast won't look right.
Meanwhile, a single source of truth not only ensures you have no data gaps but that you're using the most reliable data available (rather than using whatever nuggets you can find). As a result, you create a better forecast.
Misconception 2: Historical inventory levels don’t matter
During periods of fast growth, retail brands will typically throw historicals (past sales and inventory levels) to the wind. Why? Because when brands see exponential growth (like when ecommerce sales jumped 39% in Q1 2021), your historicals no longer seem relevant.
But even the most mature brands often only consider historical sales when forecasting – not historical inventory levels.
When supply satisfies demand, the consequences of this go unnoticed. But all it takes is one stockout to compromise your inventory forecasts.
How so? You might see no sales for a certain product on your sales sheet. But without historical inventory levels adding color, this can be misconstrued as a natural decline in customer demand (perhaps a new seasonal trend?). In reality, you didn’t have enough safety stock or you were simply out of stock.
As a result, your forecast will wildly underestimate how much to restock for that period moving forward.
Or, the opposite could be true. You could assume you were stocked out when customers simply lost interest in the SKU, leading to overordering.
Misconception 3: SKU variants should be forecasted separately
No company sells the same exact product forever. You'll eventually introduce a new version, whether it be a replacement (like when Apple releases a new iPhone) or another offering (like a new color of the iPhone).
When this happens, you might be tempted to forecast this new variant on its own. After all, it is technically a new product with its own demand curve. But without historical data, these forecasts are wild guesses at best. And they're likely to leave you overstocked or stocked out.
The better strategy is to link Version 2.0 with its predecessor. That way, you can leverage Version 1.0's historical data to increase the accuracy of both SKU variants' forecasts.
After all, neither variant sells in a vacuum. So, by linking related SKUs, you can factor in total demand (for all versions of the product) and account for product cannibalization.
Take new iPhone models, for instance. The historical data will show that sales for older versions dry up when a new model is released. But total demand for the iPhone (all versions) remains roughly the same.

Source
So, you can safely assume that you no longer need to stock older models at the same level when the next model is released – just the new model.
Similarly, when Apple introduces the existing iPhone model in a new color, you can infer that the total demand for all variants will remain roughly the same. But the new color will cannibalize some sales for older options. By linking these variants, Apple can adjust order quantities for each color offering to reflect consumer preferences.
Once each SKU variant has built its own historical data set, you can unlink them and forecast future demand for each variant separately. However, keeping them linked creates a more in-depth data set for when you introduce the next version of the product. Meaning, better forecasts straight out the gate.
Misconception 4: All channels should be analyzed together
Common sense says that if your inventory supports all your channels, you should be able to forecast for those channels together. But brands that do this rarely get their projections right.
That's because forecast accuracy is based on actual customer demand – not sales. But when channels are analyzed together, your forecasts need to assume that total sales equate to total demand. This is not always the case.
Say you sold 20,000 units via your direct-to-consumer channel every quarter for the past year. Meanwhile, your wholesale partner has ordered 10,000 units for the past four quarters. Based on this information, you'll need 30,000 units to meet next quarter's demand, right? Maybe not.
Where DTC sales directly reflect consumer demand, wholesale purchases do not. After all, that wholesaler might not be selling through as much inventory as they're ordering.
This would create a small stockpile of your products at their warehouse, driving up their unit economics. To respond, they'll likely purchase fewer units or replenish less often.
But your forecast won't see this coming. So, when this wholesale order doesn't come through as anticipated, you'll be the one left overstocked.
The smarter approach? Forecast each channel separately. Then, add each channel's inventory needs together. That way, you can factor in channel-specific data points (like your wholesale partner's sell-through rate) to increase your forecast's accuracy.
Misconception 5: Manually forecasting inventory works
Sorry to break it to you, but manual forecasting with Excel (or any comparable tool) doesn’t work. Sure, it offers you a bit more control. But it’s reactive and prone to human errors that could easily be avoided with automation.
For instance, most brands that forecast their inventory needs manually use spreadsheets. But forecasting, by definition, means looking ahead. So, why would you use a tool that’s always looking backward?
Static spreadsheets, by nature, only ever reflect a single moment in time (one that already happened). And to use it, you first have to update it.
When you finally get around to consulting that data, it’s already outdated. So, you’re reacting to whatever demand increase or supply chain issue just happened rather than working ahead to prevent it. and you waste a lot of time updating the spreadsheets to make this happen.
Not to mention that one silly human error (after all, to err is human) can skew the projections exponentially. (Just imagine forgetting a digit.)
Both these shortcomings lead teams to make decisions (like overordering) that harm their cash flow and bottom line – despite the data “supporting” their decision.
How to improve the impact of your forecasting
When it comes to these false inventory assumptions, we believe that retailers care less about the forecasts being off and more about the impact these misguided projections have on their inventory. Particularly because any overstock or understock is wildly expensive to fix.
For instance, according to a McKinsey report, retailers will need to spend $39 billion to return to pre-pandemic inventory levels after 2020 and 2021 stockouts. (This number does not include the additional costs due to brands overstocking to try and reach this goal.)
So, besides not falling victim to the common inventory misconceptions, what are other ways to improve the impact of your forecasts?
Routinely audit your inventory
Knowing where your stock levels sit is step one.
After all, your forecast might say you don't need to restock a bestselling SKU. But you know for a fact (because you just audited your inventory recently) that you're 200+ units past your replenishment point.
This shrinkage might be due to theft, returns not being processed correctly, or in-transit inventory not being tracked accurately. Whatever the cause, it’ll affect your replenishment needs all the same.
Despite your forecast, common sense would tell you to place a purchase order for more units or to set a more conservative reorder point to account for longer lead times. But you would never know that you're at risk of stocking out had you not recently audited your inventory.
Admittedly, these audits can be a massive undertaking. Of course, some tools can automate the process slightly, but you'll still need to have a human running the show. However, these audits don't always need to be a several-day (or -week, as the case may be) event.
Enter cycle counting.
What is cycle counting?
Cycle counting is a perpetual inventory auditing procedure that confirms your physical inventory counts match your inventory records. Rather than counting all your inventory in one swoop, you'll audit a small portion of stock every day. This way, you count your entire inventory over a set timeframe.
Some brands may count from the highest to lowest priced items. Meanwhile, others begin in one warehouse corner and work their way through every aisle. Both strategies ensure that all inventory is counted on a rotating basis.
Any errors discovered during these smaller counts will signal the need to investigate these discrepancies. That way, you can get ahead of what's causing it and adjust your inventory records accordingly. And as a result, your data will be more reliable, leading to smarter inventory forecasts.
Lean on an inventory forecasting tool
Adopting inventory forecasting software makes it easy to avoid those common misconceptions. That's because these tools leverage historical sales data and sales trends to effortlessly make precise predictions without introducing human errors.
Take Cogsy, for example.
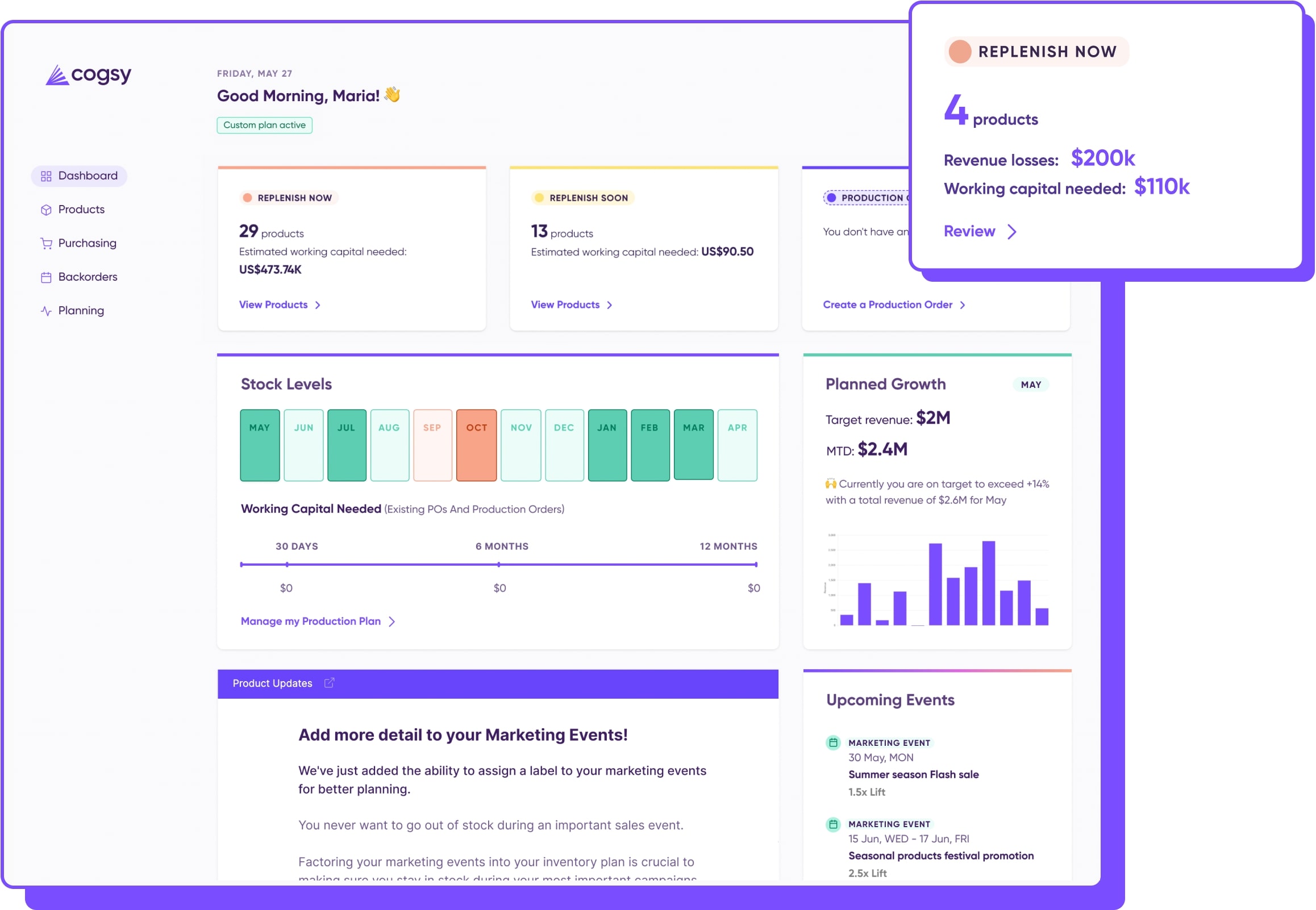
Cogsy is an inventory forecasting software that supercharges how you meet customer demand. And it's built to be the source of truth for all Shopify and Amazon brands.
With Cogsy, brands can place orders faster, reduce the frequency of stockouts, and minimize the amount of excess stock hanging out at their warehouse.
That’s because the Cogsy platform provides a godlike view of your stock levels, sales history, restock needs, incoming purchase orders, and upcoming marketing events. All in one place. So you always know how much inventory you have available (what's just sitting there and what needs to be replenished).
Cogsy then uses that data to build 12-month demand forecasts with pinpoint accuracy. You can even run "what-if" scenarios within the tool to find your best-case, worst-case, and most probable inventory strategies.
Best part? As new information becomes available, your inventory forecasts update automatically, improving your forecast accuracy.
That way, you can make smarter, more informed decisions, respond faster than your competition, and hit your most audacious revenue goals. (Brands that use Cogsy, like Caraway, generate 40% more revenue and save 20+ hours a week on average.)
Reduce your inventory risk with dropshipping
What if you could remove forecasting from the equation? You know, where it makes sense to do so – like when you're:
- Testing a new product category that doesn’t have historical data
- Adding less common sizes that tend to have less demand
- Offering bulky products that are difficult to ship or store
That's dropshipping, for you.
With dropshipping, you don't maintain inventory on-hand for your listed products. Instead, you offer a virtual inventory (or digital collection of available products). Then, when a customer makes a purchase, you fulfill that order via a third-party supplier.
In other words, dropshipped products never pass through your warehouses. This allows you to keep less inventory on hand and radically reduces your inventory risk.
(You can even manufacture those products once the order is placed. That way, you're only manufacturing what there's demand for, so you don't tie up more capital in inventory than you need to.)
Want to avoid the common forecasting mistakes with dropshipping? Reach out to the Convictional team to learn more and get started.
As co-founder and CEO of Cogsy, Adii is empowering retail brands to pursue operational excellence. Previously, Adii co-founded ecommerce website builder WooCommerce (acquired by Automattic) and ecommerce marketing automation platform Conversio (acquired by CampaignMonitor).
To Launch & Scale Your Digital Marketplace
Chat with us to learn more